Personalized Email Recommendations
Solution Background and Business Value
Improving user engagement using email has been a staple of the growth playbook for a long time. But by personalizing the content of the email campaigns you can go a step further! Personalized recommendations, when effectively implemented, have a significant impact on enhancing key business metrics such as active user counts and order rates. Even in established systems improvements in recommendation quality can lead to substantial business benefits. Kumo enables users to swiftly curate and operationalize cutting-edge recommendation models which can be used to drive email campaigns.
Data Requirements and Kumo Graph
For item-to-user recommendations we can get away with using a relatively simple dataset, but Kumo allows you to take the model much further by including additional sources of signal.
Core Tables
There are three core tables which we need:
- Users table: holds information about all users that we want to make recommendations for. It should include a
user_id
so it can be connected to each individual transaction/order event. The table can also include other user features, such as age, location, etc. we can also include userJOIN TIMESTAMP
which is taken into account when training the model. - Items table: contains information about the items available for recommending. It should include an
item_id
so it can be linked to individual transactions. Kumo also supportsSTART TIMESTAMP
andEND TIMESATAMP
columns, including such columns into the data allows Kumo models to take into account that some items were only available for a limited time, it also prevents the model from recommending items which are no longer available! The items table can also include other information about the items, such as price, color, category, etc. - Transactions/Orders table: This table records all purchase events from which the model learns to model user-item affinity. It should include the
TIMESTAMP
of the event as well asuser_id
anditem_id
. The table can also include other properties of the transaction, e.g. total amount, payment method, …
Additional Table Suggestions
Alongside above tables we can optionally include many more sources of signal, such as:
- (optional) Merchants table: Information about merchants in the marketplace.
- (optional) Search query events: What did users search for in the marketplace
- (optional) Item rating events: How did users rate items, e.g. numerical rating
- (optional) Item return events: Items returned by the users
- (optional) Comment/review events: Review data, which can include text
- (optional) Wishlist events: Recording additions of items to wish lists
- And many more possibilities!
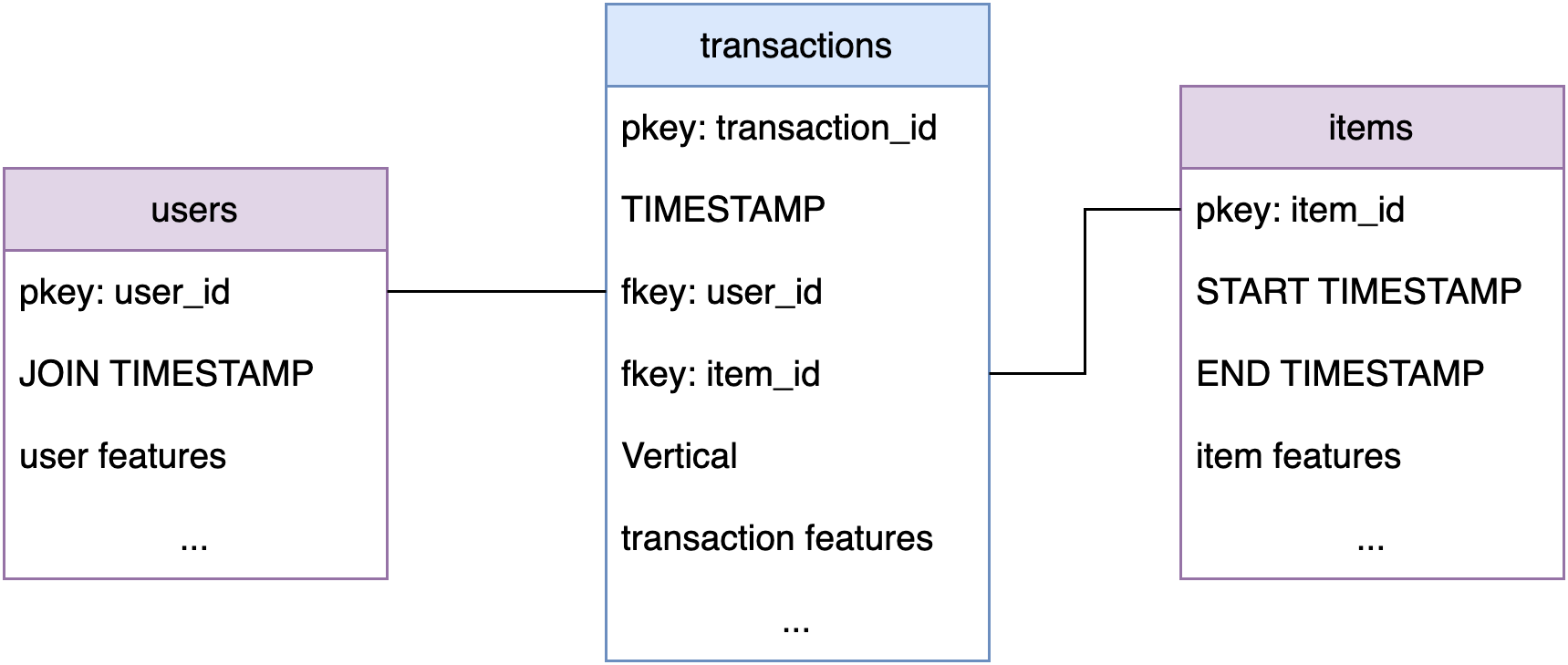
Predictive Query
We can create a recommendation model in Kumo with a very simple predictive query, which predicts the distinct list of item_id
’s each user is likely to buy in a X
day time horizon.
PREDICT LIST_DISTINCT(transactions.item_id, 0, X, days) RANK TOP 12
FOR EACH users.user_id
This predictive query learns item-user affinity by considering conversion over X
day periods, it is worthwhile experimenting with different horizons depending on the business needs (see H&M Personalization Walkthrough).
Kumo allows you to quickly experiment with many different models, for example training only on a subset of users or items, such as only email eligible users or high value items, depending on what information is available in the tables:
PREDICT LIST_DISTINCT(transactions.item_id WHERE item_id.price > 10, 0, 30, days) RANK TOP 12
FOR EACH users.user_id WHERE users.email_eligible == 1
This way you can easily create a model optimized for high value items and email eligible users. But we don’t need to limit the recommendations to the item_id
granularity. If the email campaign requires more coarse recommendations you can also recommend at the item category
, or vertical
levels and orchestrate campaigns around that:
PREDICT LIST_DISTINCT(transactions.vertical, 0, 30, days) CLASSIFY
FOR EACH users.user_id WHERE users.email_eligible == 1
Since we’re dealing with much fewer entities to recommend (item verticals instead of items) we can use the CLASSIFY
syntax and instead of receiving just the top K
recommendations, Kumo will produce a score for each possible value!
Deployment
Most email campaigns run in a batched fashion. In practice the integration would look something like this:
Each week (or other pre-specified cadence):
- Update the data in the Kumo connector path so that recommendations are produced with the freshest data
- Produce recommendations for all eligible users, and write them back to the data lake
- Use the predictions to personalize the content/recommendations in the emails
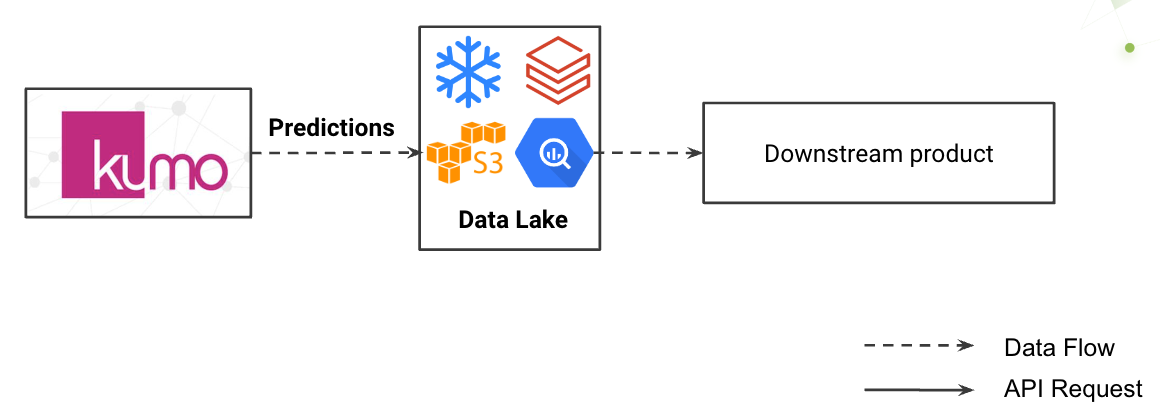
The end user experience are completely personalized recommendations, for example eligible users receive a weekly email with personalized content
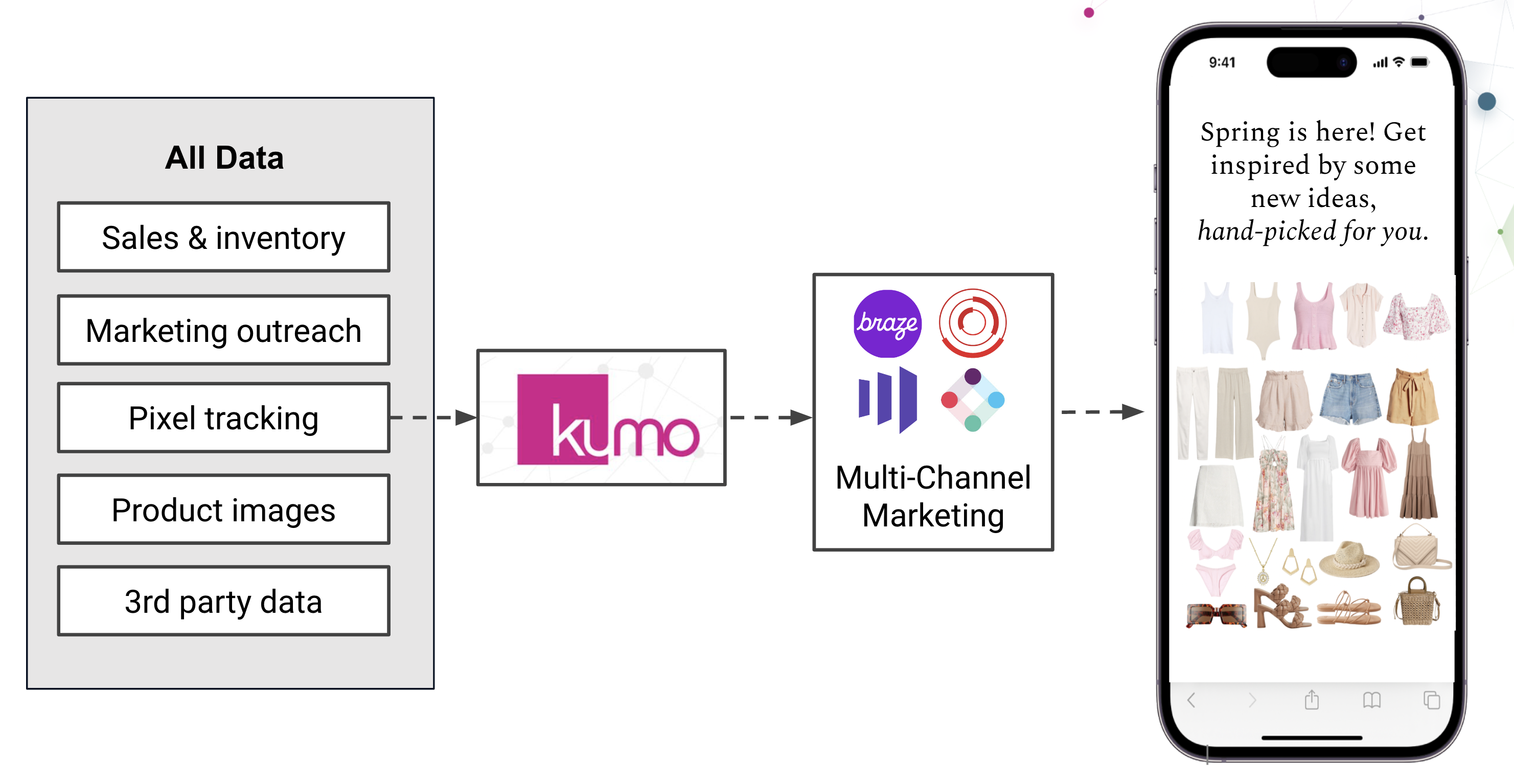
Updated 12 months ago